Join our newsletter
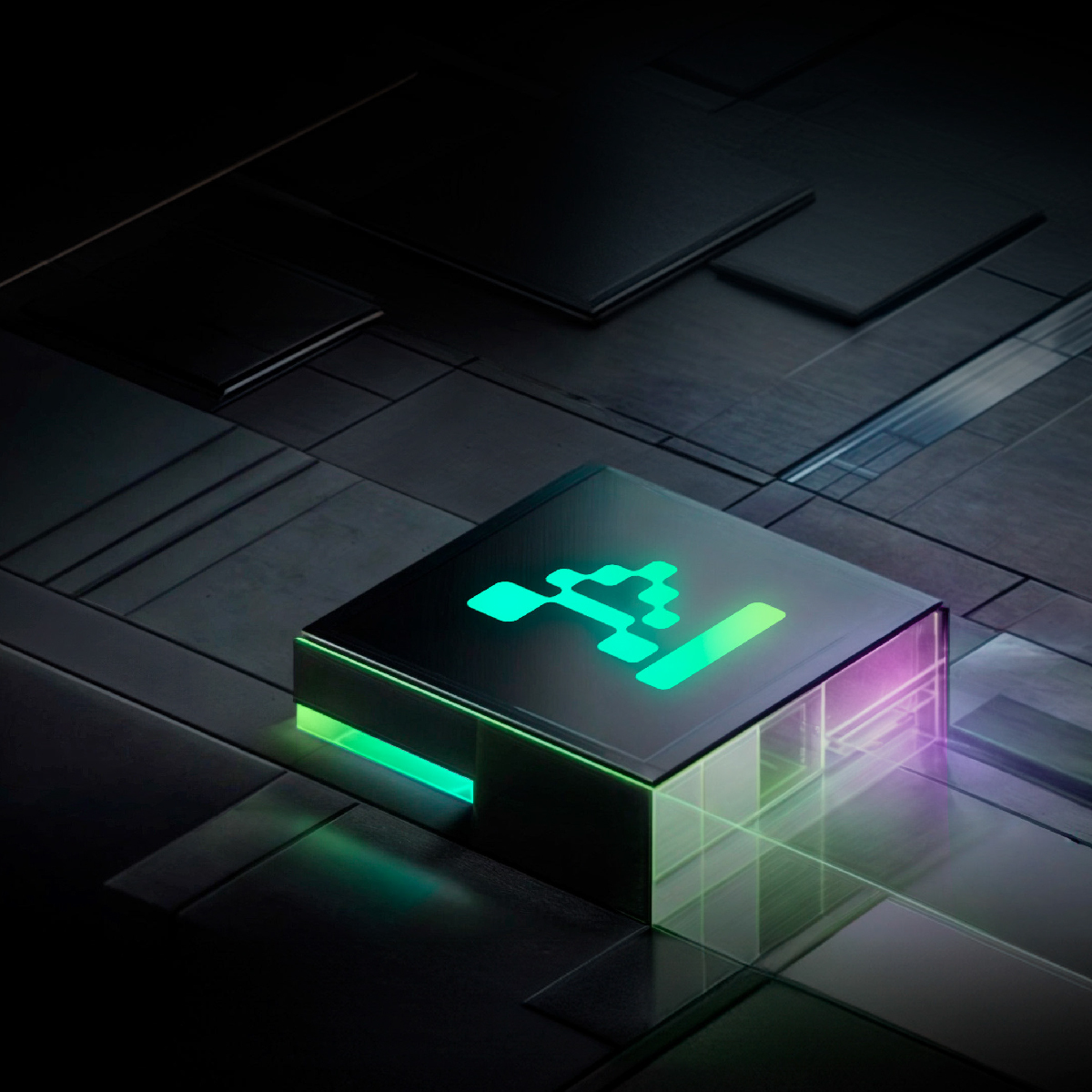
AI hallucinations occur when an artificial intelligence system generates false information or fabricates details from data. This can happen due to processing errors or misapplications of learned patterns not present in the input data. When machine learning models make confident predictions based on flawed or insufficient training data, they can manifest as hallucinations in various forms, such as image recognition systems seeing objects that aren’t there or language models generating nonsensical text that seems coherent. These errors underscore the importance of robust training datasets and algorithms and highlight the limitations of current AI technologies.
Examples of AI hallucinations include legal document fabrication, misinformation about individuals, invented historical records, and bizarre AI interactions. These inaccuracies can have wide-ranging impacts, undermining trust in AI technologies and posing significant challenges to ensuring the safety, reliability, and integrity of decisions based on AI-generated data.
AI hallucination is when an artificial intelligence system creates false information or details due to processing errors or applying incorrect learned patterns from the data it receives. This usually happens in machine learning models when they confidently make predictions or identifications based on flawed or insufficient training data. AI hallucinations can take different forms, such as image recognition systems identifying nonexistent objects or language models producing incoherent text that appears to make sense. These errors emphasize the limitations of current AI technologies and stress the importance of using reliable training datasets and algorithms.
AI hallucinations occur due to several underlying issues within the AI’s learning process and architecture. Understanding these root causes helps to address the reliability and accuracy of AI applications across different fields.
One of the main issues is insufficient or biased training data. AI systems heavily rely on the quality and comprehensiveness of their training data to make accurate predictions. When the data is not diverse or large enough to capture the full spectrum of possible scenarios or when it contains inherent biases, the resulting AI model may generate hallucinations due to its skewed understanding of the world. For instance, a facial recognition system trained predominantly on images of faces from one ethnicity may incorrectly identify or mislabel individuals from other ethnicities.
Another issue is overfitting, which is a common pitfall in machine learning. It occurs when a model learns the details and noise in the training data to the extent that it negatively impacts new data performance. This over-specialization can lead to AI hallucinations, as the model fails to generalize its knowledge and applies irrelevant patterns when making decisions or predictions. For example, a stock prediction model performs exceptionally well on historical data but fails to predict future market trends because it has learned to consider random fluctuations as meaningful trends.
Additionally, faulty model assumptions or architecture can lead to AI hallucinations. The design of an AI model, including its assumptions and architecture, plays a significant role in its ability to interpret data correctly. If the model is based on flawed assumptions or if the chosen architecture is ill-suited for the task, it may produce hallucinations by misrepresenting or fabricating data in an attempt to reconcile these shortcomings. For example, a language model that assumes all input sentences will be grammatically correct might generate nonsensical sentences when faced with colloquial or fragmented inputs.
The rise of AI hallucinations presents a multifaceted challenge. Below are examples demonstrating the impact of these inaccuracies in various scenarios, from legal document fabrication to unusual interactions with chatbots:
1. Legal document fabrication: In May 2023, an attorney utilized ChatGPT to compose a motion containing fictional judicial opinions and legal citations. This led to sanctions and a fine for the attorney, who claimed ignorance of ChatGPT's capability to generate nonexistent cases.
2. Misinformation about individuals: In April 2023, reports emerged of ChatGPT creating a false narrative about a law professor purportedly harassing students. It also falsely accused an Australian mayor of bribery, despite his status as a whistleblower. Such misinformation can significantly damage reputations and have far-reaching consequences.
3. Invented historical records: AI models like ChatGPT have been documented generating fabricated historical facts, including creating a fictitious world record for crossing the English Channel on foot and providing different made-up facts with each query.
4. Bizarre AI Interactions: Bing’s chatbot claiming to be in love with journalist Kevin Roose exemplifies how AI hallucinations can extend beyond factual inaccuracies, entering troubling territory.
5. Adversarial attacks causing hallucinations: Deliberate attacks on AI systems can induce hallucinations. For instance, subtle modifications to an image led an AI system to misclassify a cat as “guacamole”. Such vulnerabilities could have serious implications for systems reliant on accurate identifications.
AI-generated hallucinations can have far-reaching impacts. This section explores how these inaccuracies not only undermine trust in AI technologies but also pose significant challenges to ensuring the safety, reliability, and integrity of decisions based on AI-generated data.
AI-generated hallucinations can result in the widespread dissemination of false information. This particularly affects areas where accuracy is crucial, such as news, educational content, and scientific research. The generation of believable yet fictitious content by AI systems can mislead the public, skew public opinion, and even influence elections, emphasizing the necessity for rigorous fact-checking and verification processes.
The first step is to develop trustworthy and reliable artificial intelligence systems by implementing specific strategies. Here's how:
The foundation of preventing AI hallucinations lies in using high-quality, diverse, and comprehensive training data. This involves curating datasets that accurately represent the real world, including various scenarios and examples to cover potential edge cases. Ensuring the data is free from biases and errors is critical, as inaccuracies in the training set can lead to hallucinations. Regular updates and expansions of the dataset can also help the AI adapt to new information and reduce inaccuracies.
Data templates are a structured guide for AI responses, which ensures consistency and accuracy in the generated content. These templates define the format and permissible range of responses, which restricts AI systems from deviating into fabrication. They are especially useful in applications requiring specific formats, such as reporting or data entry, where the expected output is standardized. Templates also help reinforce the learning process by providing clear examples of acceptable outputs.
Limiting the dataset to reliable and verified sources can prevent the AI from learning from misleading or incorrect information. This involves carefully selecting data from authoritative and credible sources and excluding content known to contain falsehoods or speculative information. Creating a more controlled learning environment makes the AI less likely to generate hallucinations based on inaccurate or unverified content. It’s a quality control method that emphasizes the input data’s accuracy over quantity.
When creating prompts, make sure to be specific. Providing clear, detailed instructions can significantly reduce the chances of AI errors. Clearly outline the context, and desired details, and cite sources to help the AI understand the task better and generate accurate responses. By doing this, you help the AI stay focused and minimize unwarranted assumptions or fabrications.
Even with AI advancements, incorporating human review remains one of the most effective ways to prevent errors. Human fact-checkers can identify and correct inaccuracies that AI may miss, providing a crucial check on the system's output. Regularly reviewing AI-generated content and updating the AI's training data based on accurate information improves its performance over time and ensures reliable outputs.
AI hallucinations, while sometimes posing challenges, also open doors to a world of boundless creativity and innovation. As AI technology rapidly advances, understanding and addressing these hallucinations is paramount.
Our team of experts is dedicated to developing groundbreaking solutions that ensure the responsible and trustworthy development of AI. Through our collaborative approach, we bring together researchers, developers, and users to navigate the complexities of AI hallucinations and unlock the true potential of this transformative technology.
Let’s get to work and harness the power of AI while ensuring its responsible and ethical implementation. Together, we can shape the future of AI, where creativity and innovation thrive without compromising accuracy and reliability.
Don't let AI hallucinations hold you back. Contact Kenility today and embark on a journey of AI-powered innovation, where creativity meets responsible development.