Join our newsletter
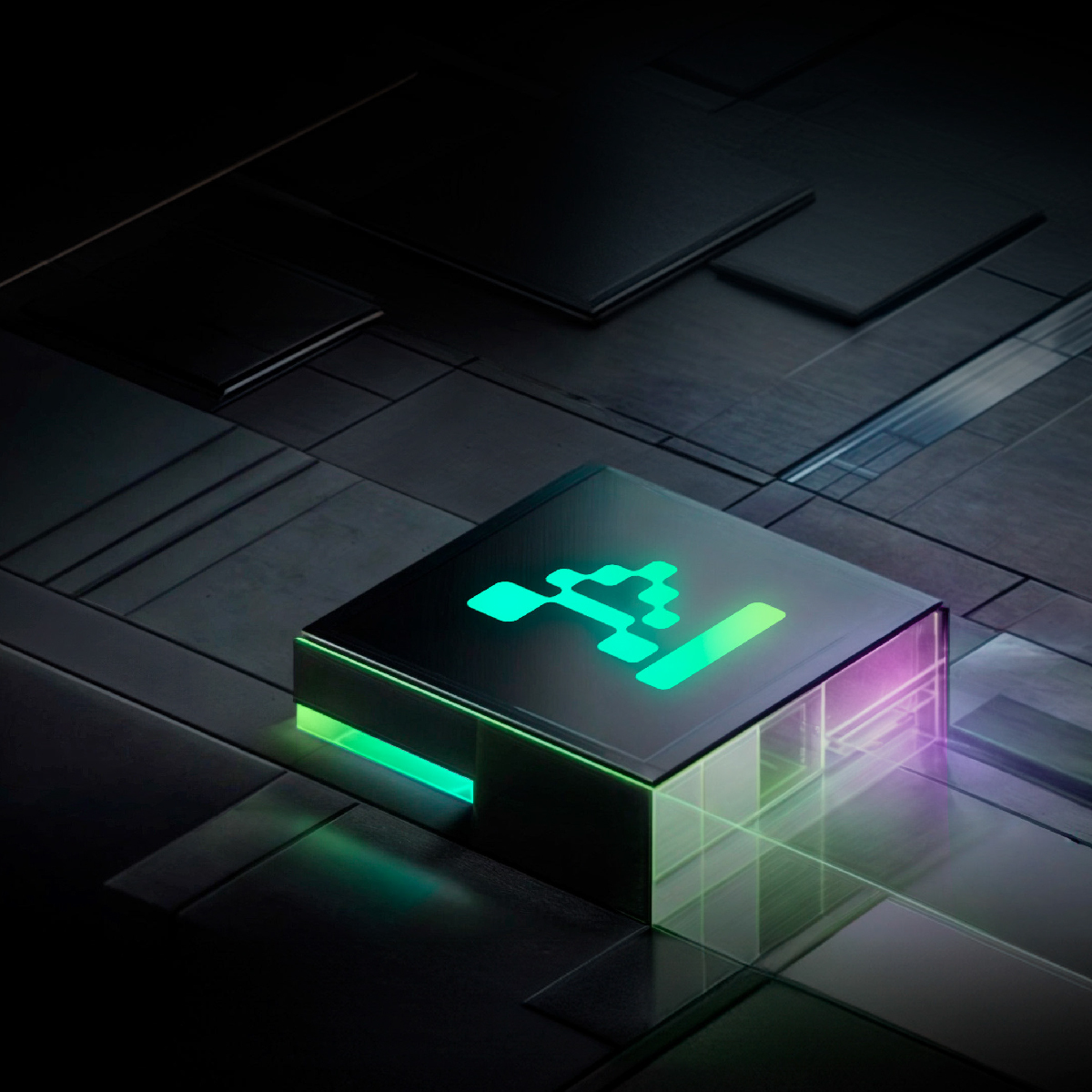
Artificial intelligence (AI) has permeated our world, transforming everything from self-driving cars to in-home voice assistants. Once relegated to science fiction, AI is rapidly becoming an indispensable part of our lives. As we approach an era of super-intelligent computers, with capabilities surpassing human analytical abilities, ethical considerations become paramount. One such concern is algorithmic bias, the unfairness inherent in the performance of AI systems, influenced by prejudices ingrained in the data they are trained on.
Similar to human biases explored in psychology, algorithmic bias arises from skewed data used to train AI systems. This data, often reflecting historical or societal inequities, can lead to discriminatory outcomes in areas like:
Bias can infiltrate AI systems in various ways:
Addressing algorithmic bias requires a multi-pronged approach:
Regulation and Standards: Governments and organizations should establish regulations and ethical guidelines to minimize AI bias.
Ignoring the issue of algorithmic bias can have profound consequences. It can:
Organizations like the Algorithmic Justice League, founded by Joy Buolamwini, are raising awareness and advocating for responsible AI development. By uniting researchers, governments, and the public, we can work towards a future where AI benefits everyone, fostering a more just and equitable society.