Join our newsletter
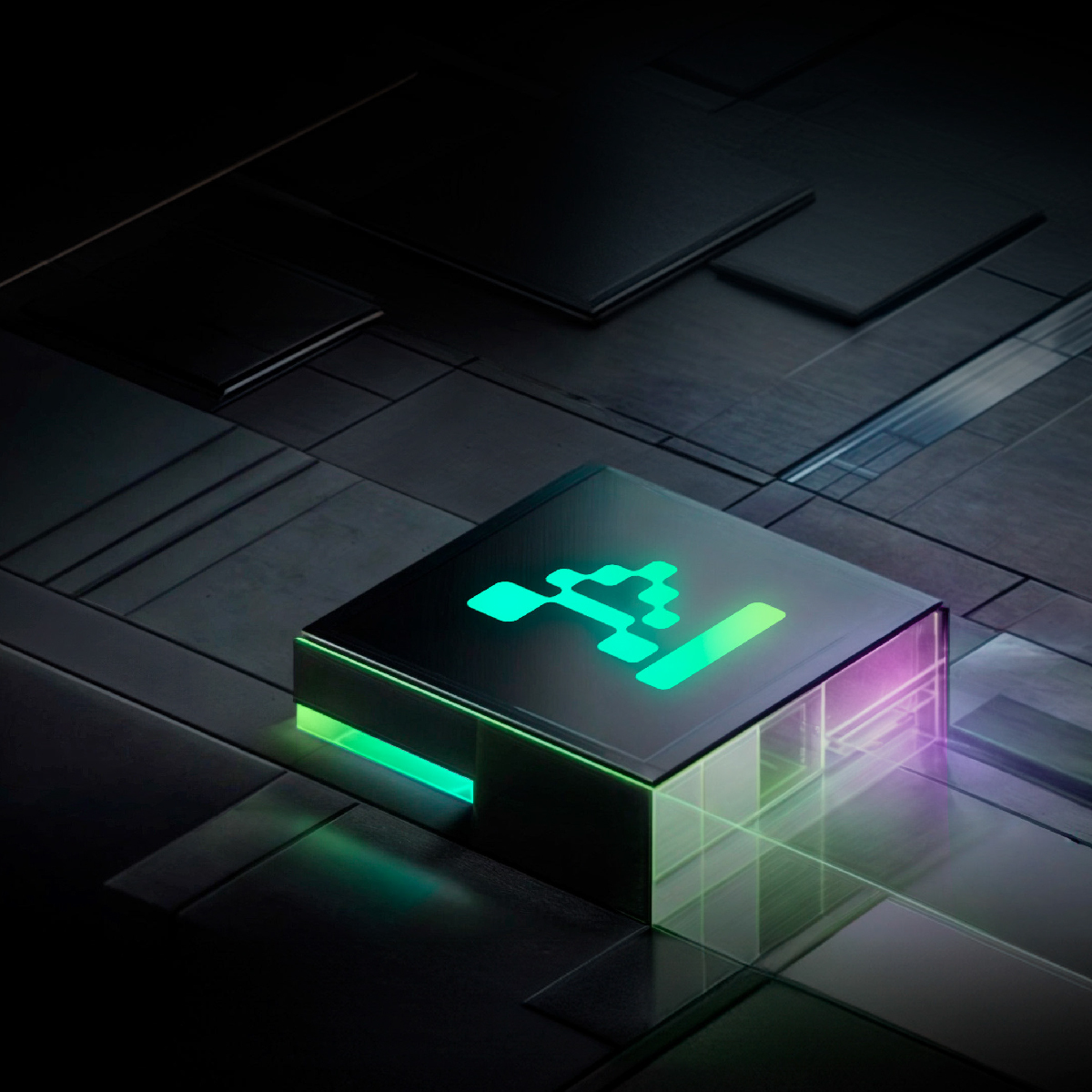
As the use of artificial intelligence (AI) increases across various industries, businesses need to manage the risks associated with its implementation. While AI can provide many benefits, such as improved efficiency and accuracy, it can also pose risks if not properly managed. In this article, we'll explore how to understand, develop, implement, and monitor an AI risk management strategy for your organization.
Before diving into the specifics of managing AI risk... It refers to the potential negative consequences that can arise from the use of AI technologies. These consequences can be caused by a variety of factors, such as errors in algorithms, lack of transparency and accountability, or unintended consequences of AI actions.
There are various types of AI risks, including technical risks, business risks, and societal risks. Technical risks refer to issues that arise from the use of AI algorithms, such as errors or biases. Business risks can include misalignment with business objectives or revenue loss. Societal risks refer to the potential negative impact on society, such as the exacerbation of social inequality or the erosion of privacy.
Some of the most common risks and challenges associated with the implementation of AI include data quality and privacy concerns, algorithmic fairness and bias, and issues related to the robustness and security of AI systems. These risks can cause harm to individuals or society as a whole, and can also result in reputational damage or financial loss for businesses.
Data quality is a significant concern when it comes to AI. AI algorithms rely on large amounts of data to make decisions, and if the data is incomplete, biased, or inaccurate, it can lead to incorrect conclusions. This can be particularly problematic in industries such as healthcare, where incorrect diagnoses can have serious consequences for patients.
Privacy is another major concern when it comes to AI. As AI systems collect and process vast amounts of data, there is a risk that personal information could be exposed or misused. This could lead to identity theft, financial fraud, or other types of harm.
Algorithmic fairness and bias is also significant challenge when it comes to AI. AI systems can inadvertently perpetuate or even amplify existing biases in society. For example, if an AI system is trained on data that is biased against certain groups, it may make decisions that disadvantage those groups.
Finally, the robustness and security of AI systems is a major concern. AI systems can be vulnerable to hacking or other forms of cyber attacks, which could lead to data breaches or other types of harm. Additionally, AI systems can be vulnerable to errors or malfunctions, which could lead to unintended consequences.
The impact of AI on businesses and society is significant and can be both positive and negative. While AI has the potential to increase efficiency and improve decision-making, it can also have unintended negative consequences. The use of AI in certain industries, such as healthcare, finance, and transportation, can create risks that need to be addressed.
In the healthcare industry, for example, AI has the potential to improve patient outcomes by enabling more accurate diagnoses and personalized treatment plans. However, there are also risks associated with the use of AI in healthcare, such as the potential for misdiagnosis or the loss of privacy.
In the finance industry, AI has the potential to improve fraud detection and risk management. However, there are also risks associated with the use of AI in finance, such as the potential for biased decision-making or the loss of jobs due to automation.
In the transportation industry, AI has the potential to improve safety and efficiency. However, there are also risks associated with the use of AI in transportation, such as the potential for accidents caused by malfunctioning AI systems or the loss of jobs due to automation.
Overall, it's important to carefully consider the potential risks and benefits of AI before implementing it in any industry or setting. By doing so, we can work to ensure that AI is used responsibly and ethically which benefits society as a whole.
Artificial Intelligence (AI) has become an integral part of many organizations, and its use is only expected to increase in the coming years. However, with the benefits of AI come new risks that organizations must manage. Developing an AI risk management strategy is crucial for any organization that is using or considering the use of AI.
The first step in developing an AI risk management strategy is to identify and assess the risks associated with AI. This can involve reviewing existing literature, identifying the potential risks associated with your specific use case, and engaging with stakeholders to understand their concerns. It is important to consider both the short-term and long-term risks associated with AI, as well as the potential impact on stakeholders.
One potential risk associated with AI is bias. AI systems are only as unbiased as the data they are trained on, and if the data is biased, the AI system will be biased as well. This can lead to unfair outcomes, such as discrimination against certain groups of people. Another potential risk is the impact on employment. AI systems have the potential to automate many jobs, which could lead to job loss for some workers. It is important to identify and assess these risks, as well as any others that may be specific to your organization.
Once risks have been identified, it's important to establish a risk management framework. This involves developing policies and processes that outline how the risks will be managed, who is responsible for managing them, and what controls will be put in place to mitigate the risks. The risk management framework should be tailored to your organization's specific needs and risks.
One control that can be put in place to mitigate the risk of bias in AI systems is to ensure that the data used to train the system is diverse and representative. This can help to reduce the risk of bias in the system. Another control that can be put in place is to establish an oversight committee to review AI systems and ensure that they are operating fairly and ethically.
An effective AI risk management strategy involves integrating risk management into your organization's culture. This can involve training employees on AI risk management, establishing clear communication channels for reporting risks, and regularly reviewing and updating your risk management policies and processes. It is important to ensure that all employees understand the risks associated with AI and their role in managing those risks.
Regularly reviewing and updating your risk management policies and processes is also important. As AI technology evolves and new risks emerge, your risk management framework should evolve as well. By regularly reviewing and updating your framework, you can ensure that your organization is effectively managing the risks associated with AI. In conclusion, developing an AI risk management strategy is crucial for any organization that is using or considering the use of AI. By identifying and assessing AI risks, establishing a risk management framework, and integrating AI risk management into your organization, you can effectively manage the risks associated with AI and ensure that your organization is operating fairly and ethically.
As the use of AI continues to grow, businesses need to establish a risk management framework to mitigate potential risks. Once a framework has been established, various techniques can be used to further reduce AI risks.
Data quality and privacy are critical issues when it comes to AI risk management. Businesses must ensure that the data used to train and test AI systems is accurate, reliable, and unbiased. This means that companies need to invest in data cleaning and validation to ensure that the data used is of high quality. Additionally, businesses must take measures to protect personal data and confidential information. This can include implementing strong encryption methods, restricting access to sensitive data, and implementing strict data retention policies.
Moreover, businesses must also ensure that the data used to train AI systems is diverse and representative of the population it is meant to serve. This will help to avoid biases in the data and ensure that the AI system is fair and inclusive.
Algorithmic fairness and bias are important considerations when using AI, as biased algorithms can perpetuate discrimination and social inequality. To mitigate this risk, businesses can use techniques such as counterfactual analysis and causal inference to identify and address bias in AI algorithms.
The counterfactual analysis involves looking at hypothetical scenarios where certain variables are changed to see how the outcome would differ. This can help businesses to identify where bias may be present in their algorithms and make changes to address it. Causal inference, on the other hand, involves identifying causal relationships between variables to better understand the impact of different factors on the outcome.
Ensuring the robustness and security of AI systems is essential to mitigating the risk of system failure or cyber-attacks. Techniques like adversarial testing can be used to identify vulnerabilities in AI systems and improve their resilience to attacks. Adversarial testing involves intentionally exposing an AI system to malicious attacks to identify weaknesses and improve its security.
Additionally, businesses must ensure that their AI systems are regularly updated and maintained to address any potential vulnerabilities. This can include implementing regular security audits, patching any vulnerabilities, and providing regular training to employees on best security practices.
Overall, implementing these techniques can help businesses to mitigate potential AI risks and ensure that their systems are secure, fair, and effective.
Continuous monitoring and evaluation of AI risk is crucial to ensure that the risk management strategy remains effective over time. This can involve: Continuous monitoring of AI performance.
Continuous monitoring of AI performance can help identify potential risks early on. This can be done using techniques such as real-time monitoring, anomaly detection, and predictive analytics.
Auditing the AI risk management strategy can help identify areas for improvement and ensure that the strategy remains effective. Audits should be conducted regularly and should involve a review of the risk management policies and processes, as well as an assessment of their effectiveness.
The landscape of AI risks and regulations is constantly evolving, and businesses must be prepared to adapt to these changes. This can involve keeping up to date with new regulations, engaging with stakeholders to understand emerging risks, and updating risk management policies and processes as needed.
Managing AI risk is a critical consideration for businesses that are using or considering the use of AI. By understanding the nature of AI risk, developing a risk management strategy, implementing risk mitigation techniques, and monitoring and evaluating the risk management strategy over time, businesses can minimize the potential negative consequences of using AI and ensure that its implementation is both safe and effective.